Machine Learning: The Secret Sauce of Precision Agriculture for Fighting Crop Diseases
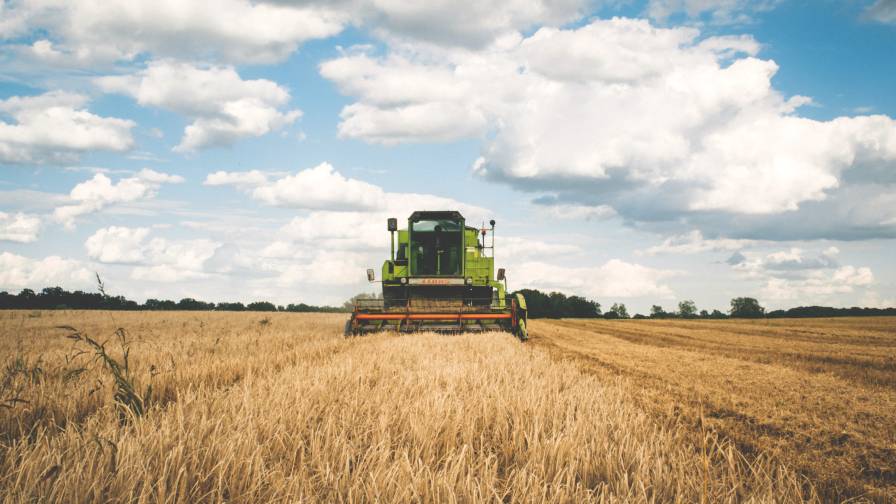
The advancement of machine learning applications for agriculture has come at the right time to offer a different approach comparatively to the traditional ways for disease detection. Photo credit: Pexels
With the development of agricultural technologies, crop and plant diseases have been advancing as well, causing degradation of both quality and quantity of agricultural products. While methods for curing these diseases do exist, they are often costly, dangerous for the environment, and for the consumer.1 From there, it doesn’t come as a surprise that early-stage detection of diseases became a priority for many farms, aiming at quality and yield losses reduction.
The advancement of machine learning applications for agriculture came in a right time to offer a different approach comparatively to the traditional ways for disease detection. Constant monitoring of farms either by farmers, agronomists, or crop consultants ends up being expensive and time consuming as it must be continuous to see the changes. One might argue that on small farms there is no need for machine learning algorithms or drones, however even when you think you know all about your farm, not everything can be seen with a human eye.
Easy acquisition of vast amounts of data and fast processing options are additional boosts for enabling the new detection approaches to be more optimal. It is all about predicting, targeting, and saving money. To put it into perspective, deep learning, a subset of machine learning2, can take years of the crop data related to diseases and crop performance. It can structure the algorithms in layers to create an artificial ‘neural network’ to make decisions on its own, and eventually produce a probability model. Having that type of information helps the stakeholders to increase the disease detection accuracy in comparison to the human eye visual inspection.
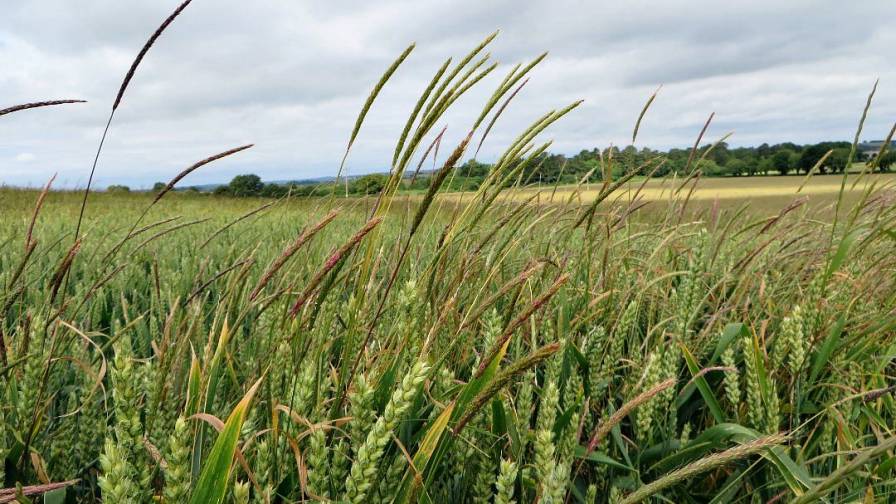
Black-grass emerging within winter wheat. Photo credit: Laura Crook
Crop diseases and pests vary through geographic regions and climates, like Fusarium head blight, wheat and rice blast, yellow rust, black-grass, only to name a few. While they differ in what crops they attack and when, as well as what symptoms they show in the crops, the implications they have on global food production and food security are the same and great.
MORE BY BARBARA HORVATIC
To put some scientific severity on this claim: on average crop diseases account for 42% of the production losses of the six most important food crops.3 Crop losses in particular due to pests and pathogens can be direct, but indirect as well. And it is the indirect effects like harvest and postharvest quality, and possible accumulations of toxins that should not be underestimated4 and bring an even bigger emphasis on early disease detection and prediction models.
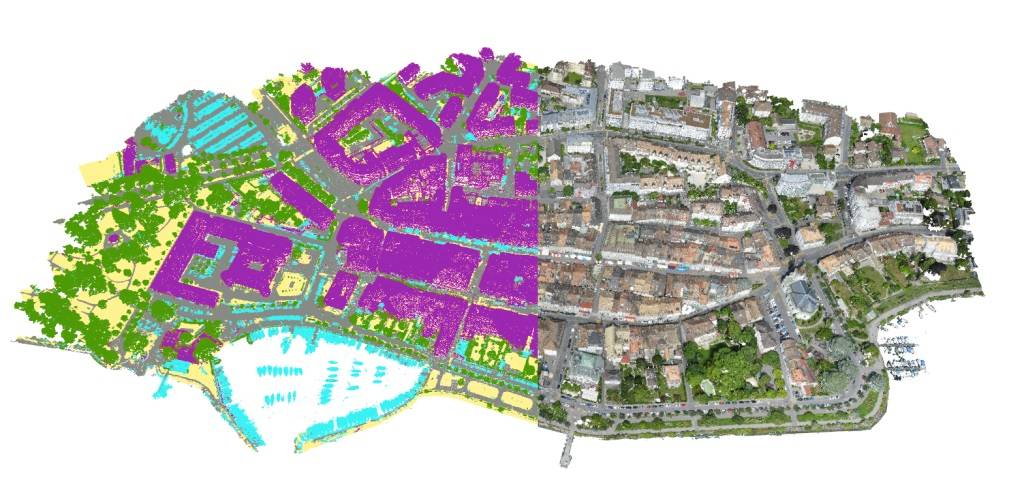
Many companies have started using machine learning and invested into research and development teams to create new applications which will help stakeholders in decision making in agriculture industry. Photo credit: Pix4D
The applicability of machine learning in agriculture has many benefits, from aforementioned disease detection, pest detection, and plant breeding, to water conservation and real-time predictions. Many companies have started using machine learning and invested into research and development teams, Pix4D included, to create new applications which will help stakeholders in decision making in agriculture industry.
Pix4D globally, believes in the ability of photogrammetry and machine learning techniques to revolutionize today’s workflows and to enable many new ones.
As a final thought, while majority of us recognizes food production and security are important challenges to tackle, perhaps we should also acknowledge we do live in a time of advanced technologies and that each new machine learning algorithm might just be a step closer into making these challenges, a little bit less of a challenge.